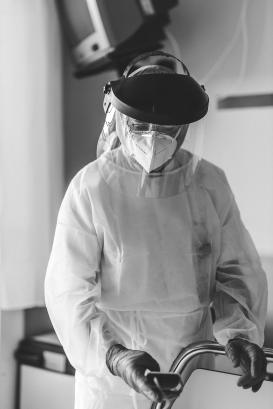
The paper titled "Unravelling COVID-19 social mortality patterns using conditional inference tree algorithm" is authored by Lisa Cavillot (Sciensano), Laura Van den Borre (Sciensano), Katrien Vanthomme (Ghent University), Aline Scohy (Sciensano), Patrick Deboosere (BRISPO), Brecht Devleesschauwer (Sciensano and Ghent University), Niko Sailing the Waves of European Public Health: Exploring a Sea of Innovation (Lisbon, Portugal).
The study examined sociodemographic and socioeconomic patterns associated with COVID-19 mortality in Belgium during 2020, focusing on how different factors influenced COVID-19 deaths, deaths from other causes (OCOD), and differences between these. Using logistic regression and conditional inference tree analysis, the authors found that older age, male gender, living in collectivities, lower education levels, and socioeconomic deprivation significantly increased the odds of dying from COVID-19. For individuals not in collectivities, low education emerged as a key predictor. Living in collectivities was consistently the strongest predictor of COVID-19 mortality across age groups, underlining the importance of preventive measures in these settings.
Full text is available here
Citation: Cavillot, L., Van den Borre, L., Vanthomme, K., Scohy, A., Deboosere, P., Devleesschauwer, B., ... & Gadeyne, S. (2024). Unravelling COVID-19 social mortality patterns using conditional inference tree algorithm. European Journal of Public Health, 34(Supplement_3), ckae144-1003.